COVID-19 prognostic modeling using CT radiomic features and machine learning algorithms: Analysis of a multi-institutional dataset of 14,339 patients: COVID-19 prognostic modeling using CT radiomics and machine learning
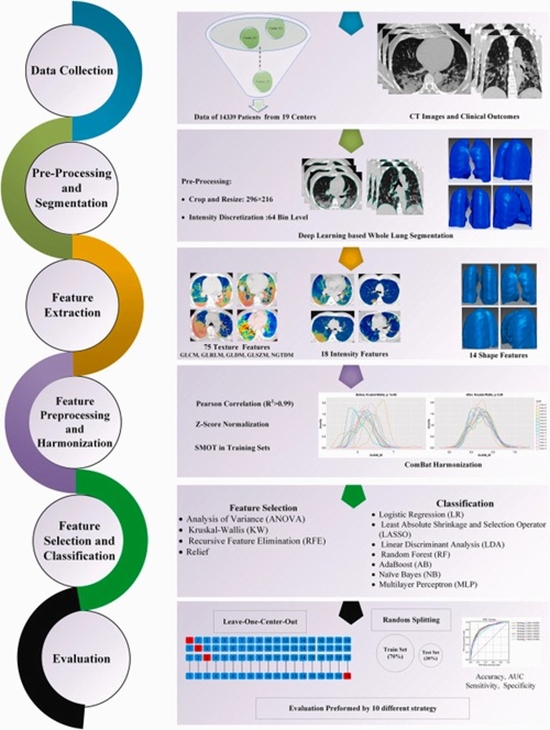
Background: We aimed to analyze the prognostic power of CT-based radiomics models using data of 14,339 COVID-19 patients.
Methods: Whole lung segmentations were performed automatically using a deep learning-based model to extract 107 intensity and texture radiomics features. We used four feature selection algorithms and seven classifiers. We evaluated the models using ten different splitting and cross-validation strategies, including non-harmonized and ComBat-harmonized datasets. The sensitivity, specificity, and area under the receiver operating characteristic curve (AUC) were reported.
Results: In the test dataset (4,301) consisting of CT and/or RT-PCR positive cases, AUC, sensitivity, and specificity of 0.83 ± 0.01 (CI95%: 0.81-0.85), 0.81, and 0.72, respectively, were obtained by ANOVA feature selector + Random Forest (RF) classifier. Similar results were achieved in RT-PCR-only positive test sets (3,644). In ComBat harmonized dataset, Relief feature selector + RF classifier resulted in the highest performance of AUC, reaching 0.83 ± 0.01 (CI95%: 0.81-0.85), with a sensitivity and specificity of 0.77 and 0.74, respectively. ComBat harmonization did not depict statistically significant improvement compared to a non-harmonized dataset. In leave-one-center-out, the combination of ANOVA feature selector and RF classifier resulted in the highest performance.
Conclusion: Lung CT radiomics features can be used for robust prognostic modeling of COVID-19. The predictive power of the proposed CT radiomics model is more reliable when using a large multicentric heterogeneous dataset, and may be used prospectively in clinical setting to manage COVID-19 patients.
نظر دهید